Artificial Intelligence (AI), Machine Learning (ML), Deep Learning (DL), and Generative AI are often used interchangeably, but they stand for distinct entities within the AI ecosystem. Understanding the differences and specific use cases of these technologies is crucial for gaining a comprehensive perspective on AI. This essay explores the key differences between AI, ML, DL, and Generative AI, and discusses their unique applications.
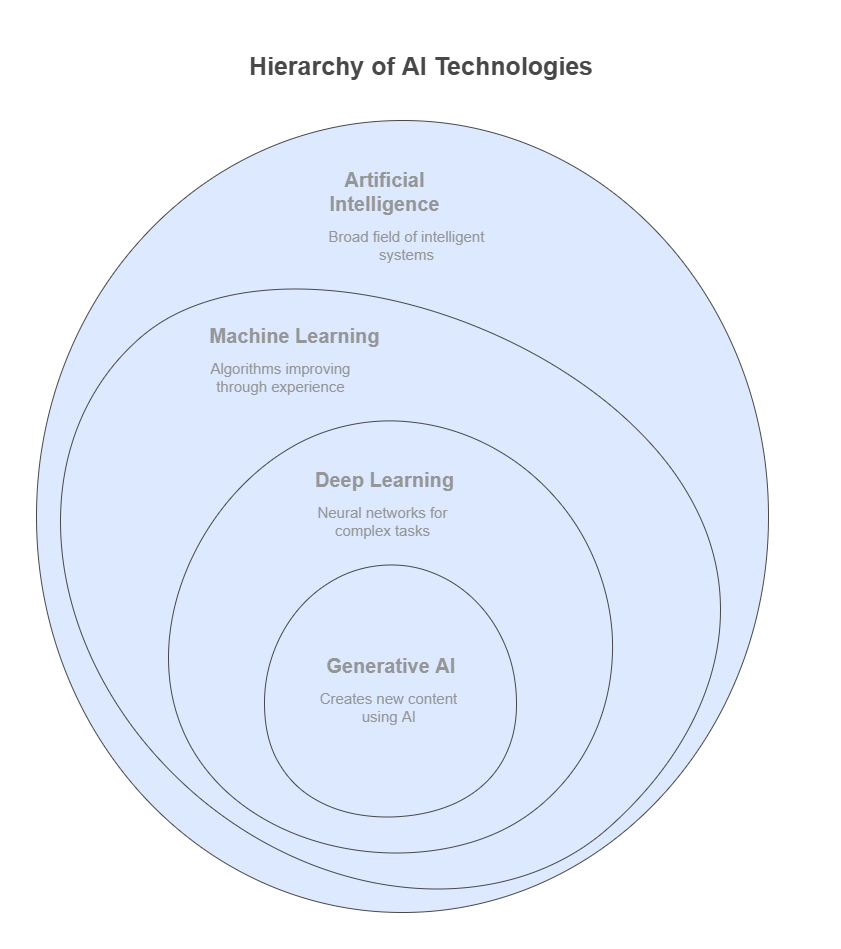
Also Read: 12 Important AI Terms that You Must Know
Getting to Know the Basics
Concept | Description |
Artificial Intelligence (AI) | A broad field of computer science focused on creating systems capable of performing tasks that typically require human intelligence, such as reasoning, learning, problem-solving, perception, and language understanding. Includes subfields like machine learning, natural language processing, robotics, and more. |
Machine Learning (ML) | A branch of AI is based on the idea of designing algorithms that allow machines to learn from data and make decisions. Primarily classified into supervised, unsupervised, and reinforcement learning. |
Deep Learning (DL) | A subset of ML focuses on using multiple layers of artificial neural networks to process large quantities of data, learning to recognize complex patterns. Excel in applications like image and speech recognition. |
Generative AI | Algorithms that create new content such as text, images, and audio. Examples include Generative Adversarial Networks (GANs) and transformers. Capable of generating realistic and original content. |
Key Differences
Aspect | AI | ML | DL | Generative AI |
Scope and Purpose | Encompasses the entire AI technology spectrum | Develops algorithms to learn from data | Uses deep neural networks to analyze and interpret complex data patterns | Generates new content based on learned data distributions |
Complexity and Data Requirements | Varies from simple systems to advanced neural networks | Requires significant data for effective models | Needs large amounts of labeled data and high computational resources | Requires extensive datasets for training, computationally intensive |
Learning and Adaptation | Can include learning and non-learning systems | Focuses on data-driven learning without explicit programming | Learns through multiple layers of neural networks, understanding intricate patterns | Uses learning algorithms to understand data and generate new content |
Use Cases
Artificial Intelligence (AI): In healthcare, AI systems help in disease diagnosis, patient outcome prediction, and personalized treatment plans. This revolutionizes patient care by allowing more accurate and timely interventions.
In customer service, AI-powered chatbots answer customer inquiries instantly, improving support efficiency and customer satisfaction. In finance, AI algorithms detect fraudulent transactions, manage investments, and automate trading processes, ensuring security and efficiency in financial operations.
Machine Learning (ML): Machine learning algorithms are being widely applied within a recommendation system by analyzing a person’s activity towards a recommendation to be generated that can consist of products or services, including Netflix or even Amazon, wherein the algorithms could predict failure through machine learning model-based forecasting on equipment breakdown time and so reducing downtime; for spam filtering to be successfully and efficiently deployed ML plays a core part in detection.
Deep Learning (DL): DL is really good at image and speech recognition, which powers facial recognition applications, virtual assistants like Siri and Alexa, and automated transcription services. Another critical application is Natural Language Processing (NLP), where DL algorithms enable language translation, sentiment analysis, and the development of chatbots that can understand and generate human language.
Besides this, DL is also an important part of the autonomous vehicle that processes sensor data to navigate and make real-time decisions, propelling the growth of self-driving cars.
Generative AI (Gen AI): Generative AIis transformative in the creation of content. Models, such as GPT-3, can produce human-like text, write code, and even compose creative writing pieces. In terms of image generation, GANs create photorealistic images, opening new applications in the arts, designs, and entertainment. Generative AI also composes original music, providing new tools for musicians and producers to explore and create innovative compositions.
Future Trends and Considerations
As the status of AI, ML, DL, and Generative AI continues to advance, several trends will shape their development and application. One significant trend is the integration and collaboration of different approaches in AI. Thus, machine learning and deep learning would be combined to produce more efficient and diversified systems as they would leverage each other’s strengths.
This approach of a hybrid model will improve the accuracy and performance of AI applications while making them more robust and versatile. Interdisciplinary collaboration among AI researchers, domain experts, and ethicists will be crucial as well. It is essential to solving interdisciplinary problems in complex societally related issues by ensuring responsible ethical AI deployment.
By interdisciplinary approaches, diverse perspectives are brought together, and such collaborations can assure innovation while raising ethical considerations.
One of the most important considerations in developing AI technologies concerns ethical and societal impacts. Making sure AI systems are fair and unbiased involves changing things that occur in training data as well as algorithm design. Techniques that identify the sources of biases should be developed so that AI systems can provide equitable results for everyone using them.
Another aspect is protecting user data and privacy, especially with the growing integration of AI technologies into daily routines. Implementing strong data protection measures and transparency in the use of data can foster trust and security with AI applications.
Enhanced personalization is another new trend. AI-driven solutions can offer personalized experiences in healthcare, education, and entertainment tailored to the needs and preferences of the users. Personalization can improve user satisfaction and engagement by providing services that are more relevant and effective.
Advancements in Generative AI are expanding the boundaries of creativity and innovation. The new generation of AI is also known as Generative AI, capable of creating content that has quality in many areas, such as art or scientific research. Novel and realistic content can open new possibilities for creative expression and discovery by these AI models.
For example, Generative AI may assist artists in producing unique artwork, help researchers in generating hypotheses, and design products. Still, as such technologies advance, technological progress should be balanced with responsibility toward ethics. The responsible use of Generative AI can prevent its potential misuse and ensure that its benefits are realized across society.
As these trends and considerations guide the future of AI, it is essential to balance technological advancement with ethical responsibility. By addressing ethical and societal implications, fostering interdisciplinary collaboration, and enhancing personalization, AI technologies can have a positive impact on society.
Ensuring that AI systems are fair, unbiased, and secure will build trust and pave the way for a future where AI enhances human capabilities and responsibly drives innovation.
Conclusion
AI, ML, DL, and Generative AI are the different layers of the artificial intelligence landscape. Each has unique characteristics and applications. AI is the parent field that covers all intelligent systems. ML is the field of learning from data. DL is a subset of ML applying deep neural networks to learn complex patterns, while Generative AI specializes in creating new content.
This will help us in making us understand much more about how such technologies are changing the world of business and daily life. So, the future AI and everything that can do something more than humans will depend on these technologies’ progress and how they will eventually integrate with the ethical factors surrounding them.