Artificial Intelligence (AI) is becoming an integral part of our world. With more than 60% of businesses adopting AI in some form, this technology is transforming industries and lives.
AI refers to systems that mimic human intelligence to perform tasks like understanding speech, making decisions, and analyzing data. As its influence grows, understanding key AI terms is essential for staying informed and relevant.
Here are 12 crucial AI concepts everyone should know to navigate this evolving landscape.
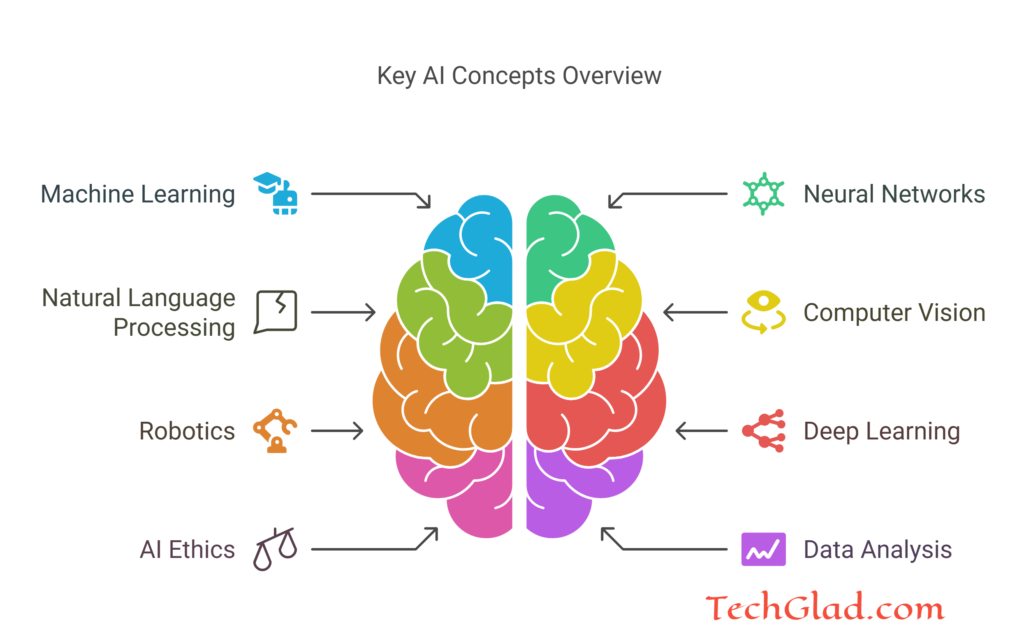
Foundational AI Concepts
1. Machine Learning (ML)
Machine learning is a sub-domain of artificial intelligence that enables computer systems to learn from available data and improve their operations without explicit programming. While it analyzes data, the system identifies patterns and relies on them to make predictions or decisions.
For example, spam filters identify patterns in the email to block unwanted spam, and recommendation systems analyze what people are doing to recommend products or movies or music that go according to individual preferences.
This technology is changing the future of healthcare, finance, marketing, and many more similar sectors by providing innovative yet efficient solutions. In health care, machine learning serves as an assistant in medical diagnostics with the help of analyses from patient data.
Within finance, it plays a serious role in detecting fraudulent behavior. It refines targeted marketing in terms of campaigns reaching the exact target. As our dependency upon data increases, so too does the importance of machine learning in driving AI advancements, hence real-world applications, as well.
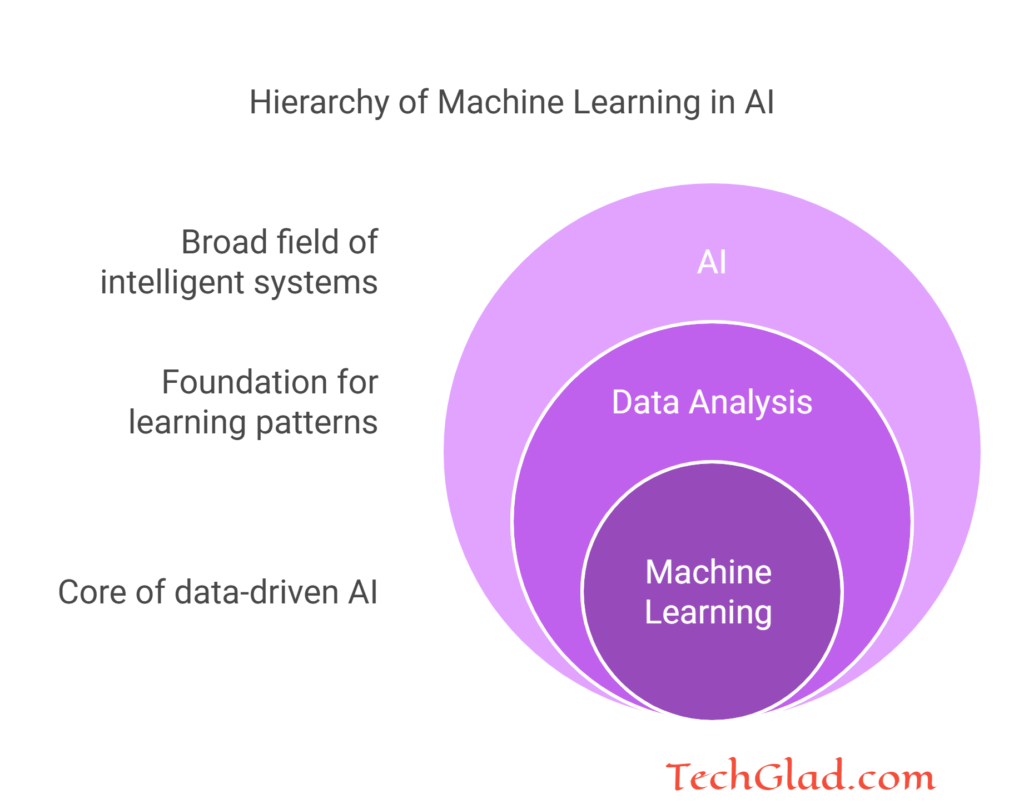
Machine Learning Basic Level Interview Questions Quiz (Multiple Choice)
2. Deep Learning (DL)
Deep learning is a special form of machine learning in the field, that applies multilayer neural networks for processing such enormous, complex data forms such as images, text and audio files. It powers images with a recognition system that recognises what is present in that photograph, or Natural Language Processing with chatbots. A strong human-like ability to mimic and learn has made it an important aspect of innovative modern AI in areas including medicine to autonomous vehicles.
3. Neural Networks
As inspired by the model of the human brain, neural networks make up layers of nodes or “neurons” that further process information.
These networks constitute the backbone for deep learning applications, permitting AI to analyze patterns in data, categorize data, and predict outcomes. Specific types exist, too, such as Convolutional Neural Networks (CNNs) for imaging and processing, and RNNs for sequence analyses. Neural networks are very critical in most systems, such as voice assistants and facial recognition technologies.
Important AI Algorithms
4. Linear Regression
Linear regression is a statistical algorithm used for predicting outcomes based on input variables. For instance, it can forecast sales by analyzing advertising spend. Despite its simplicity, it is widely used in business forecasting. However, linear regression assumes a direct, linear relationship between variables, making it less effective for complex data patterns.
5. Decision Trees
A decision tree represents a series of conditions with their decisions graphically; it is easy to read and understand. For instance, the firm can use decision trees in determining how to segment the customer in terms of their buying behavior. They are direct and hence useful for small sets but will overfit the data. Hence, it lowers accuracy when dealing with bigger data sets.
6. SVM:
Support Vector Machines are powerful algorithms used in classification and regression tasks. SVMs are strong in classification, such as whether a given image contains a dog or a cat. They are suitable for high-dimensional datasets but are computationally expensive and have significant data volume.
Understanding AI Data
7. Big Data
Big Data refers to the high volume of structured and unstructured data that is generated in enormous amounts every day coming from various sources such as social media platforms, sensors, financial transactions, and connected devices.
This data feeds into the AI systems, which enables them to train the models, find patterns, and make predictions.
Examples range from streaming platforms like Netflix recommending shows based on what big data indicates about specific people’s preferences to financial institutions spotting fraudulent transactions. However, big data requires advanced tools and technologies to process and analyze the data effectively. Hadoop and Spark are examples of such systems that help in the management and interpretation of such data to support AI-driven decision-making. The quality of big data is just as important as its volume. Poor-quality or biased data can have a profound effect on AI outcomes, including the possibility of inaccurate predictions and flawed systems.
For instance, discriminatory data in recruitment tools might lead to further discrimination, and poor data in medical applications may lead to false diagnoses. Organizations must ensure the integrity, diversity, and accuracy of the data they use. With the world generating more data each day, proper management and usage of big data become critical for the success of AI systems in any industry.
8. Data Mining
Data mining is the process of sifting through big data to determine meaningful patterns, trends, and relationships.
It transforms raw data into actionable insights, hence enabling business decisions based on that data.
For instance, retail shops will use data mining to try to understand the purchases and make appropriate stock, whereas banks do transaction data and predict a customer’s need. All these processes put together incorporate statistical methods, machine learning techniques, and database systems, all aimed at analyzing the data efficiently. Advances like SQL and specialized software make data mining a hallmark of modern business analytics.
Despite its advantages, data mining has its problems, mostly on the issue of privacy and security of the data. The extraction of insights often necessitates sensitive personal information, an ethical issue. Mishandling or misuse of the data can lead to breaches of trust, regulatory fines, and reputational damages.
To this end, businesses must adhere to data protection regulations such as GDPR while implementing strong security measures in place. Ethical practices form the bedrock of having data mining work for businesses while respecting individual privacy rights.
9. Data Annotation
Data annotation is the process of assigning labels to datasets in preparation for training AI models.
This process enables AI systems to learn and classify data correctly.
For instance, an annotator may assign a label like “dog” or “cat” to images to train a system to distinguish between the two. In text annotation, sentences are categorized for sentiment analysis, such as marking phrases as positive, negative, or neutral. Accurate annotation is key in establishing high-performance AI models; especially in applications like face recognition, autonomous vehicles, or voice assistants.
But the most labor-intensive and expensive way in which data annotation has been so far is to be implemented by humans. Usually, an enormous amount of time goes in checking the correctness of each label, mainly in terms of large data volumes. In medical imaging, or sometimes in legal document processing, the annotation even calls for subject-matter expertise.
Companies are looking for ways to reduce costs without sacrificing accuracy by outsourcing annotation tasks or using semi-automated tools. Despite these challenges, data annotation remains a foundational step in building effective AI systems.
AI Applications and Impacts
10. Natural Language Processing
NLP is a capability that equips machines to understand, interpret, and respond to human language. It powers the tools used in chatbots, voice assistants, and translation apps. It allows AI systems to generate meaningful responses by breaking down text into patterns, improving communication between humans and machines. Future improvements in NLP will create more intuitive and context-aware systems.
11. Computer Vision
Computer Vision allows machines to “see” and analyze visual data like images and videos. This technology enables self-driving cars to navigate streets and healthcare systems to identify diseases from medical scans. Despite its potential, computer vision still faces challenges in complex environments, such as identifying objects in low-light conditions.
12. Robotic Process Automation (RPA)
RPA employs AI to automate mundane tasks, such as data entry, invoice processing, or scheduling. This technology is revolutionizing industries by making processes more efficient and accurate. But with its growth comes the issue of potential job displacement, especially for roles that are repetitive in nature.
Conclusion
Those are 12 basic terms in AI; equipping you to freely participate with the AI technology world. Since AI becomes highly significant, keeping abreast with what this science is becoming a new era in everyday industry operations, it goes deep without its presence to which knowledge should be very strong on how to proceed, better, and most certainly well for what is present to explore with the modernized concept.
I just started learning artificial intelligence. This article is a good start for me. Thanks !